What is Analysis of Variance (ANOVA)?
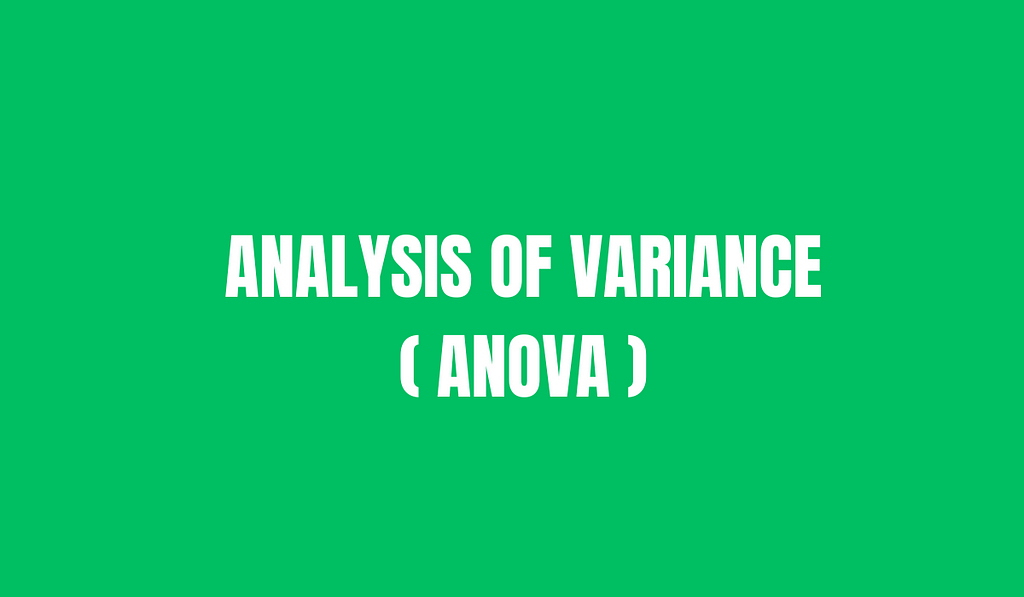
Analysis of variance (ANOVA) helps to test for differences among three or more population means. The statistician Ronald Fisher developed ANOVA in 1918. ANOVA offers a statistical test for determining whether two or more population means are equal. Therefore, it extends the t-test beyond two means. Another popular name for ANOVA is fisher analysis of variance and is the extension of t- and z-tests. It is a statistical method which is based on the law of total variance. Basically, it separates observed variance data into different parts to use for additional tests.
It divides observed aggregate variability within a data collection into two main parts:
- Systematic Variables
- Random Factors
Random factors have no statistical impact on the given data set, but systematic factors do.
The null hypothesis (H0) of analysis of variance says that there are no differences in group means. The alternative hypothesis (Ha) says that at least one group differs greatly from the overall mean of the dependent variable.
The following is the formula for ANOVA:
Summary
F=MSE/MST
where:
- F = ANOVA coefficient
- MST = The mean sum of squares because of treatment
- MSE = The mean sum of squares because of error
The overall test of equality of group means is provided by ANOVA. The analysis of variance also has the ability to regulate the overall type I error. Since analysis of variance is a parametric test, it is quite powerful.
General Assumptions
The following are some assumptions made by ANOVA:
- The data should be unrelated to one another. There should be no bias in the observations.
- The dependent variable’s values should have a normal distribution.
- There should be variance homogeneity. I go into more detail about this in this article.
Types of Analysis of Variance
The following are the different types of analysis of variance:
- One-way ANOVA
- Two-way ANOVA
Let us understand them better.
One-way ANOVA
A one-way ANOVA employs one independent variable. It examines the means of different groups to see if any of them are statistically different. This test tests the null hypothesis.
When should you use it?
The following are some assumptions made by a one-way ANOVA, and if you meet these assumptions, you know you should use it.
- There must be at least one category independent variable. In that categorical independent variable, there should be at least two or more categorical independent groups.
- There should be no correlation between the observations in each group or between the groups, suggesting that the observations are independent.
- Determine your dependent variable’s interval or ratio level. Quantitative dependent variables are necessary.
- There should be no significant outliers.
- Your dependent variable should be roughly normally distributed for each category of the independent variable.
- The variations should be homogenous. Homogeneity of variance refers to the idea that the population variances of two or more samples are equal. It supports both T tests and F tests.
One major disadvantage of using the one-way ANOVA is that, while it will tell you that at least two groups differed from each other, it will not tell you specifically which groups differed. Speaking about JUST the analysis of variance overall, the fact that it demands that the population distributions be normal and that there be homogeneity of variance is one of ANOVA’s drawbacks.
Two-way ANOVA
A two-way ANOVA helps to estimate the mean of a quantitative variable based on the levels of two categorical variables. It employs two independent variables, as opposed to one in the one-way analysis of variance. It is the key difference between one-way analysis of variance and two-way analysis of variance. The assumptions of a two-way analysis of variance are the same as those of a one-way ANOVA, except that it employs two or more categorical independent variables.
Three null hypotheses are tested simultaneously using a two-way ANOVA with interaction. The following are the null hypotheses:
- There is no difference in group means regardless of the level of the first independent variable.
- There is no difference in group means regardless of the level of the second independent variable.
- The influence of one independent variable is independent of the effect of the other independent variable.
However, when we talk about a two-way ANOVA without interaction, it tests both 1st and 2nd hypotheses but not the 3rd one. If you’re wondering what interaction implies here, it indicates that the impact of one variable is influenced by the level of the other variable.
If you are felling disappointment, read this.
Relationship between ANOVA and F-test
If you read my previous article on t-tests and f-tests, you must be familiar with f-tests and understand what they mean, what assumptions they make, and so on. But why am I bringing it up in this article? When there are three or more groups, one-way analysis of variance uses f-tests to statistically analyze the equality of means.
An f-test is used to determine whether or not a group of means are fully equal. However, in order to do so, we must use the proper ratio variances.
The f-statistic ratio for one-way ANOVA is the following:
F = between-groups variance divided by within-groups variance
When calculating the between-groups variance, the one-way ANOVA method calculates the average of each of the groups. In other words, the greater the variance in the numerator, the farther the groups are from the global mean. When the group means are further apart or separated, it becomes obvious and clear that they are different. Within-groups variance is a measurement of the distance between each data point and the group mean.
When the null hypothesis is true, F-statistics is the ratio of two variances that are close to the same value, resulting in an F-statistics value near to 1.
In a low F-value graph, the group means pile closer together than the within-group variability. At this stage, it’s hard to prove whether the graph’s groups are genuinely different at the population level.
A high F-value graph, on the other hand, demonstrates that the group averages are more evenly distributed than the variance within groups. Here, it’s obvious that the groups in the graph differ from each other at the population level.
More content at PlainEnglish.io.
Sign up for our free weekly newsletter. Follow us on Twitter, LinkedIn, YouTube, and Discord.
What is Analysis of Variance (ANOVA)? was originally published in Artificial Intelligence in Plain English on Medium, where people are continuing the conversation by highlighting and responding to this story.
https://ai.plainenglish.io/what-is-analysis-of-variance-anova-4ac72f7acc2c?source=rss—-78d064101951—4
By: Gunjan
Title: What is Analysis of Variance (ANOVA)?
Sourced From: ai.plainenglish.io/what-is-analysis-of-variance-anova-4ac72f7acc2c?source=rss—-78d064101951—4
Published Date: Fri, 21 Jul 2023 04:16:59 GMT
Did you miss our previous article…
https://e-bookreadercomparison.com/understanding-agi-implications-for-future-technology-and-software-development/